Nadine Pichelot, VP Finance at Anaplan outlines the steps finance leaders need to take to impliment machine learning into their organisations.
Recent years have seen significant developments in emerging technologies and machine learning (ML) is at the forefront of the technology revolution. It has now penetrated almost every industry across the world, and in some cases is revolutionising business processes by providing breakthroughs in complex calculations involving large data volumes.
Although the term ML is a little less known than artificial intelligence (AI) in the mainstream world, there are some recognisable examples of it in use today. From voice assistants that refine data from previous interactions to create a tailored experience, to the social media platforms that we use every day, which are consistently using machine learning to monitor our behaviour and provide us with personalised news feeds. But some of the truly innovative examples of ML lie beneath the surface. Revenue management solutions used in hotels, for example, now often incorporate ML technology into an algorithmic engine to help produce pricing and inventory recommendations.
As such, industries and organisations that aren’t yet using the technology to its full potential are placing a huge focus on incorporating it into their working practises, and the world of finance is one of them. There is continuous chatter regarding the ways that ML can improve future financial planning and analysis (FP&A) and wealth management processes, and most importantly, how finance leaders can prepare to deploy advanced analytics within their organisations.
Creating opportunities with Machine Learning
ML is incredibly versatile and can be applied to a number of different technologies to achieve a variety of objectives – but the one common denominator in the finance world is data. Ultimately, ML is just one piece of a larger analytics puzzle that includes predictive analytics, artificial intelligence, and data mining.
For finance, ML can provide FP&A teams with more opportunities to uncover and analyse business drivers from external business ecosystems and internal data that can help finance leaders make – and influence – more insightful business decisions.
How well do you really know your competitors?
Access the most comprehensive Company Profiles on the market, powered by GlobalData. Save hours of research. Gain competitive edge.
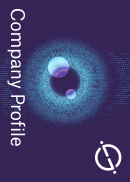
Thank you!
Your download email will arrive shortly
Not ready to buy yet? Download a free sample
We are confident about the unique quality of our Company Profiles. However, we want you to make the most beneficial decision for your business, so we offer a free sample that you can download by submitting the below form
By GlobalDataWealth management technology platform firm Forwardlane, for example, is using AI and ML technology to help wealth managers synthesise, analyse and organise the data that they are continuously drawing on in real-time to make informed decisions for their customers.
Key considerations before working alongside machines
Adopting new technologies can be a huge investment for any company, big or small. So it’s vital that they are able to truly reap the benefits of the investment and ensure they get the biggest bang for their buck. As such, there are a number of points to consider before jumping head first into an ML transformation journey:
- Is your data clean? – Financial organisations, especially those relating to wealth management roles, are built on a foundation of data, but when in such large volumes, it can get messy. Before implementing any ML technology, organisations need to realise the importance of data cleansing across all business units.
- Has your organisation implemented data governance? – During the data cleansing process, it is inevitable that organisations will come across a number of inaccuracies and errors. When this happens, finance teams should focus their attention on fixing them as a matter of urgency before any technology is implemented in order to maintain data integrity.
- How familiar are you with external, ambiguous, and unstructured data? – Get to know your data and start to explore how external indicators can help deliver better insight to the business. This is precisely where advanced analytics and ML technologies can help.
- Do you have the right tools in place to facilitate Connected Planning? – With so much data spread across multiple business units, it’s important that companies are able to effectively collaborate across teams. To do so involves adopting dynamic enterprise planning technology and collaborative business processes that can link business units across the enterprise with one another in real time. With such planning technology and business processes in place, ML technology that is implemented can better generate insights from one function, such as the supply chain, and inform an adjacent function, such as finance.
- Do you allocate enough time for reconstructing end-to-end business planning logic? – Establishing and implementing a fresh approach to business planning logic takes time and isn’t something that should be rushed, so it’s important that businesses establish a realistic time-frame to achieve this and challenge the use of lift-and-shift strategies.
- How do you plan to build capabilities for better business partnering? – Transformation is an all-encompassing term, it isn’t simply about automating processes and introducing analytics. The wider team also needs time in their schedule to be able to implement and execute their own changes, so another major focus should be adopting technologies that can best support these initiatives.
As much as we are consistently faced with scaremongering thoughts that machines will soon replace the human workforce, the truth is that they will always require human input.
As finance organisations look to embrace machine learning technologies, one of the most important considerations is to be prepared for situations in which human input will be required.
Looking to the future, emerging technologies including advanced analytics and machine-learning will augment human judgment in ways that we cannot foresee today. But finance leaders need to start preparing for that future now.